On Artificial Intelligence: ‘Existing biases that were already present are now just being replicated.’
For all its potential to transform our lives in positive ways, Artificial Intelligence can also perpetuate the biases that lead to discriminatory behaviors and policies.
This was among the cautionary tales told during the recent discussion, Bias in Technology: The Causes, Consequences, and Possible Solutions, presented by the Warren B. Rudman Center for Justice, Leadership & Public Service and the Franklin Pierce Center for Intellectual Property. Panelists included Mailyn Fidler, Assistant Professor of Law at UNH Franklin Pierce School of Law; Alexis Shore Ingber, Postdoctoral Research Fellow at the University of Michigan School of Information, and James T. McKim Jr., long-time high-tech entrepreneur and respected thought leader on diversity and digital transformation. The discussion was moderated by Laura Knoy, the Rudman Center’s Community Engagement Director.
This event was part of the Alison Curelop Series in Ethics, Professionalism and Civility.
To watch a video of the full event, filmed by ConcordTV, visit here. Quotes in this piece have been edited slightly for clarity.
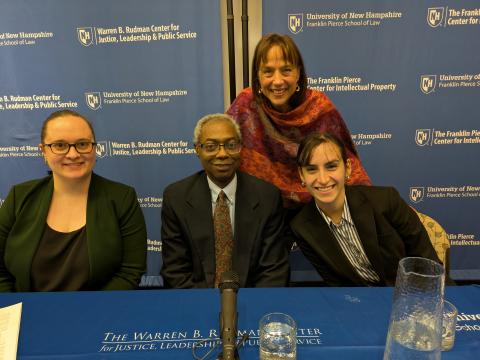
Mailyn Fidler, James T. McKim Jr., Laura Knoy (standing), Alexis Shore Ingber
As James McKim explained, while displaying an ergonomic mouse designed for right handers, bias in technology is not new and involves hardware and software as well
as AI. “Who's this biased against? Left-handed people,” he said.
Yet the speed and reach of AI systems, such as generative AI, can propagate bias in unprecedented ways.
“At a high level, we're perpetuating existing human biases or existing inequities in the world,” said Alexis Shore Ingber. “This also exists in terms of making decisions about who gets hired for a job, who gets particular medical diagnoses, who gets sentenced to jail, who gets into college. And so the existing biases that were already present are now just being replicated.”
The 400 types of biases that have been identified are divided into four categories, said McKim: cognitive bias, meaning how we think and make decisions; social attributional biases, or how we think about other people and groups of people; memory biases, meaning how we remember things; and perception about physical biases, or how our bodies interact with the world.
Mailyn Fidler proposed another way to look at bias – as simply the favoring of one outcome over another. The effects can be discriminatory, even if that’s not the intention, she said. A company may create algorithms aimed at minimizing its risk when granting loans, for instance, but the outcome can be discriminatory. “I think most people aren't setting out to build discriminatory algorithms, but they are setting out to build biased algorithms, very clearly stated biased algorithms, and those have discriminatory impacts,” Fidler said.
An algorithm can also be designed to minimize racial and gender discrimination, she said. “In this case, there's actually going to be a bias towards that over other things that the algorithm could be prioritizing, but it reduces discrimination overall,” she said.
Among the challenges in addressing discriminatory bias: Trade secrets allow companies to keep certain information, including data sets used in algorithms, from public view. “We could reform intellectual property laws, but that’s a big ask,” said Fidler. “The better thing to do here is to place legal duties of care on software developers to ensure their outcomes are within a certain acceptable range of things -- so, using tort-inspired principles, placing legal duty of care on them.”
Still, panelists agreed, certain remedies are within reach. “We are seeing some really exciting things happening at the state level,” Ingber said. “California just passed a slew of AI related laws, one of which demands transparency about how these algorithms are being created, which is going to be enacted in 2026. So that's exciting. We also have seen the Colorado AI Act, which similarly wants to see what's going on in training data,” she said.
Also important, said Fidler: incorporating a range of perspectives in developing technology.
McKim encouraged consumers to take initiative in holding tech companies accountable. “You can ask questions of the developers or the manufacturer of the product: What have you done about minimizing the negative impact of bias?” The manufacturer may not initially respond, he said. “But if enough people ask, then the company will see something. They might or might not acquiesce, but if you can submit those requests, saying ‘We found this error. We found this bias. Help us,’ hopefully, they'll want to help you, because otherwise you can give them a bad name.”
Ingber encouraged realistic expectations. “Bias is universal. It's historic. It exists in humans. It will continue to exist in humans and in technology forever. There's no way to just remove any sort of bias completely,” she said. “But we can recognize disparate impacts both at the human level and at the technology level and do more research and development that centers those folks that are disproportionately negatively impacted by bias. We can make sure that our algorithms are being built with people in mind who are often forgotten. And I think that's maybe the first step.”